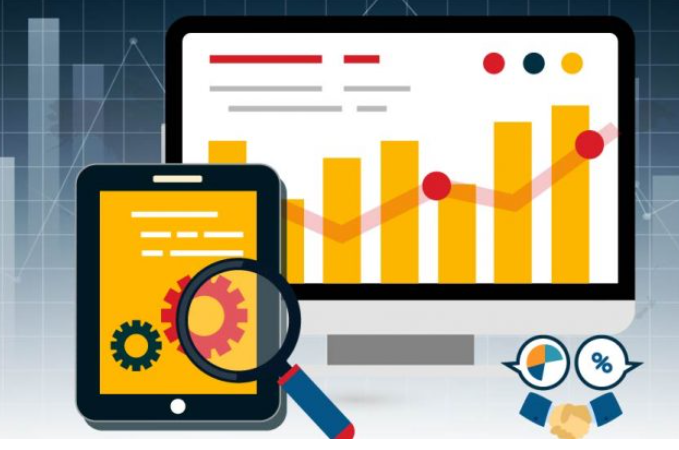
Demand forecasting is an important part of market and demand analysis, as it provides critical insights into the potential demand for a product or service over a specific time period. Naïve forecasting models are a type of demand forecasting model that are simple and easy to implement, but may be less accurate than more sophisticated forecasting techniques.
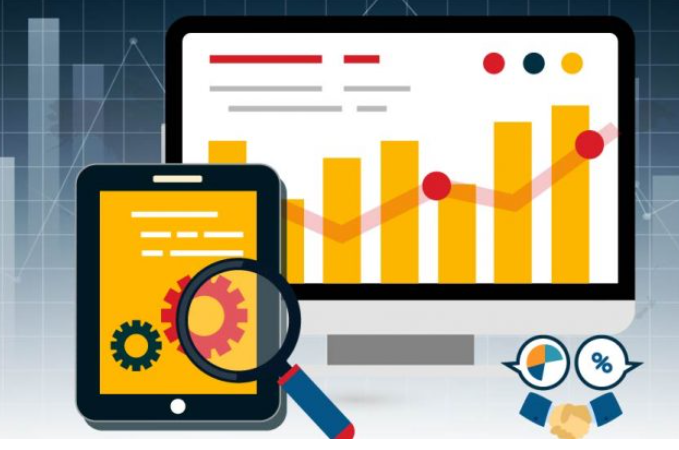
Naïve models are based on the assumption that the demand for a product or service will remain constant over time. This means that the forecast for the next period is simply equal to the actual demand in the previous period. There are several types of naïve models, including the following:
- Naïve model 1: The forecast for the next period is equal to the actual demand in the current period.
- Naïve model 2: The forecast for the next period is equal to the actual demand in the same period of the previous year.
- Naïve model 3: The forecast for the next period is equal to the average of the actual demand over the previous n periods.
Naïve models are easy to use and require minimal data preparation, which makes them a popular choice for organizations that have limited historical data or do not have the resources to implement more sophisticated forecasting techniques. However, they may be less accurate than more sophisticated forecasting techniques, as they do not take into account the many factors that can influence demand for a product or service, such as changes in consumer preferences, economic conditions, or competition.
Despite their limitations, naïve models can provide a useful benchmark for forecasting accuracy, and can be a useful starting point for organizations that are just beginning to implement demand forecasting. They are also useful for providing a quick estimate of demand, which can be useful in situations where a quick decision is needed.
Naïve models
Naïve forecasting models are based exclusively on historical observation of sales (or other variables such as earnings, cash flows, etc). They do not explain the underlying casual relationships which produces the variable being forecast.
Advantage: Inexpensive to develop, store data and operate.
Disadvantage: does not consider any possible causal relationships that underlie the forecasted variable. 1.To use actual sales of the current period as the forecast for the next period; then,
Yt+1 = Yt
2. If we consider trends, then, Yt+1 = Yt + (Yt – Yt-1)
3. If we want to incorporate the rate of change, rather than the absolute amount; then,
Yt+1 = Yt (Yt / Yt-1)
Demand Forecasting : Smoothing techniques
Smoothing techniques
Higher form of naïve models:
A. Moving average: are averages that are updated as new information is received. With the moving average a manager simply employs, the most recent observations, drops the oldest observation, in the earlier calculation and calculates an average which is used as the forecast for the next period.
Limitations:
One has to retain a great deal of data.
All data in the sample are weighed equally.
B. Exponential smoothing: uses weighted average of past data as the basis for a forecast.
Yt+1 = aYt + (1-a) Yt or Y new = a Y old + (1-a) Y’ old, where,
Y new = exponentially smoothed average to be used as the forecast
Y old = most recent actual data
Y’old = most recent smoothed forecast
a = smoothing constant
Smoothing constant (or weight) has a value between 0 and 1 inclusive.
Register & Download PDF for Educational Purposes Only
Project Planning and Management Study notes for M. plan Sem-II

project planning and management.pdf (Size: 1.29 MB / Downloads: 29)
Register as member and login to download attachment [pdf] by right-click the pdf link and Select “Save link as” use for Educational Purposes Only
Disclaimer
Information on this site is purely for education purpose. The materials used and displayed on the Sites, including text, photographs, graphics, illustrations and artwork, video, music and sound, and names, logos, IS Codes, are copyrighted items of respective owners. Front Desk is not responsible and liable for information shared above.